Human in the Loop Document Automation
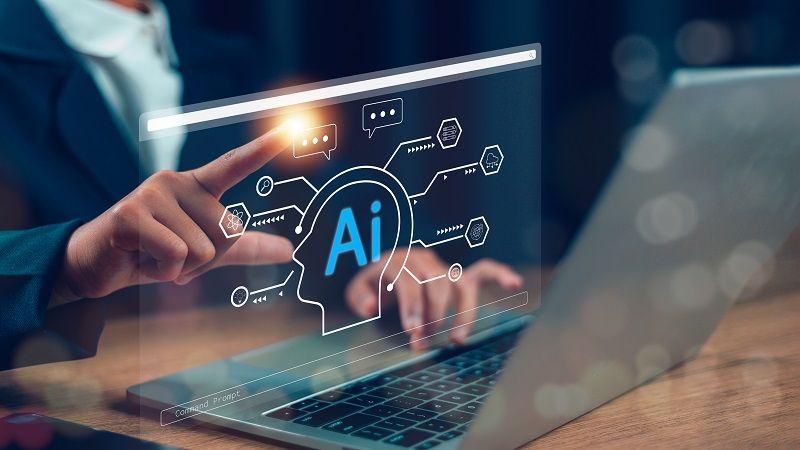
AI
document automation
human in the loop
Challenge - Underwriting Knowledge is not captured
In the insurance industry, underwriting is a critical yet complex process that requires specialized knowledge and experience. Traditionally, this underwriting knowledge is passed down from one employee to another through on-the-job training and documented in process guidelines. However, this approach often leads to knowledge gaps and inefficiencies, as valuable insights and nuances can be lost when experienced underwriters leave the company.
For a leading insurance provider, this knowledge retention challenge was hindering their ability to streamline and automate underwriting processes effectively. Without a comprehensive repository of labeled data capturing the intricacies of underwriting decisions, introducing machine learning-based automation was not feasible.
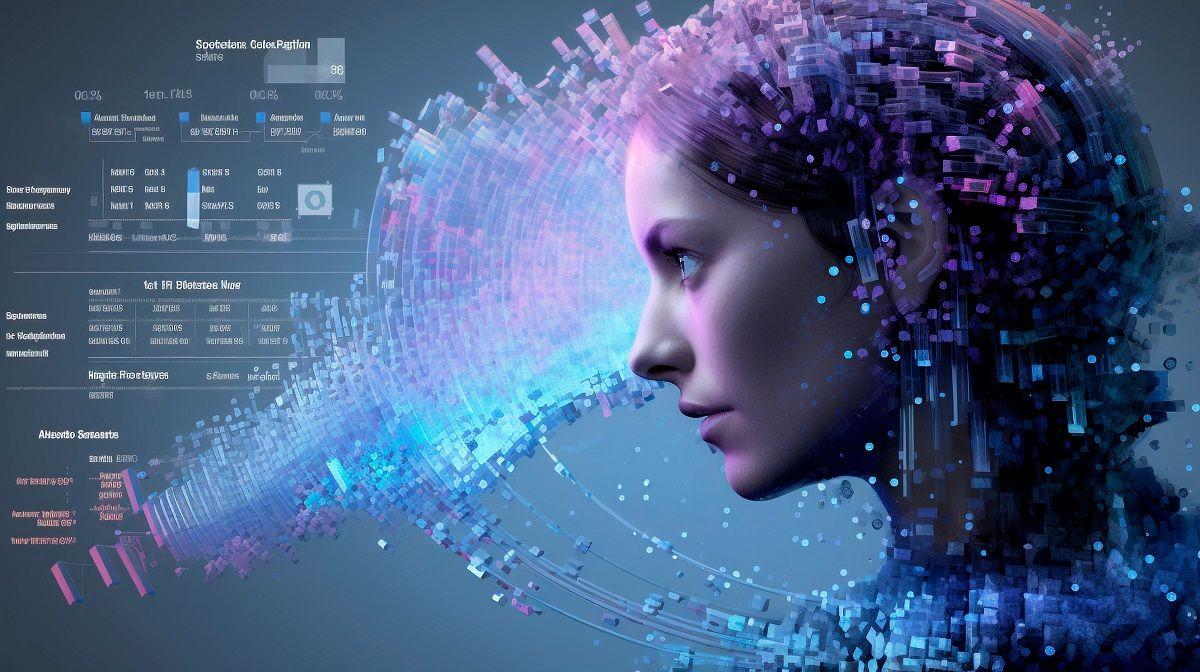
Approach - Instrument Processes to Annotate Data
To address this challenge, the project team introduced a web-based annotation tool that seamlessly integrates with underwriters' existing workflows. As underwriters review submissions and documents, the tool prompts them to annotate relevant fields and data points, similar to how Google uses Captchas for human verification.
This human-in-the-loop approach allows underwriters to effortlessly contribute their expert knowledge while performing their regular tasks. The annotated data is then used to train machine learning models for entity extraction, classification, and decision support, enabling more effective automation of underwriting processes.
Key Features and Benefits
- Seamless Integration: The annotation tool is designed to fit naturally into underwriters' existing processes, minimizing disruption and ensuring high adoption rates.
- Knowledge Capture at Scale: By leveraging the collective expertise of underwriters across the organization, the solution captures and consolidates underwriting knowledge at an unprecedented scale.
- Continuous Learning: As underwriters annotate more data over time, the machine learning models continuously improve, enabling higher levels of automation and decision support.
- Knowledge Retention: The captured underwriting knowledge is systematically documented and retained, mitigating the risk of knowledge loss due to employee turnover.
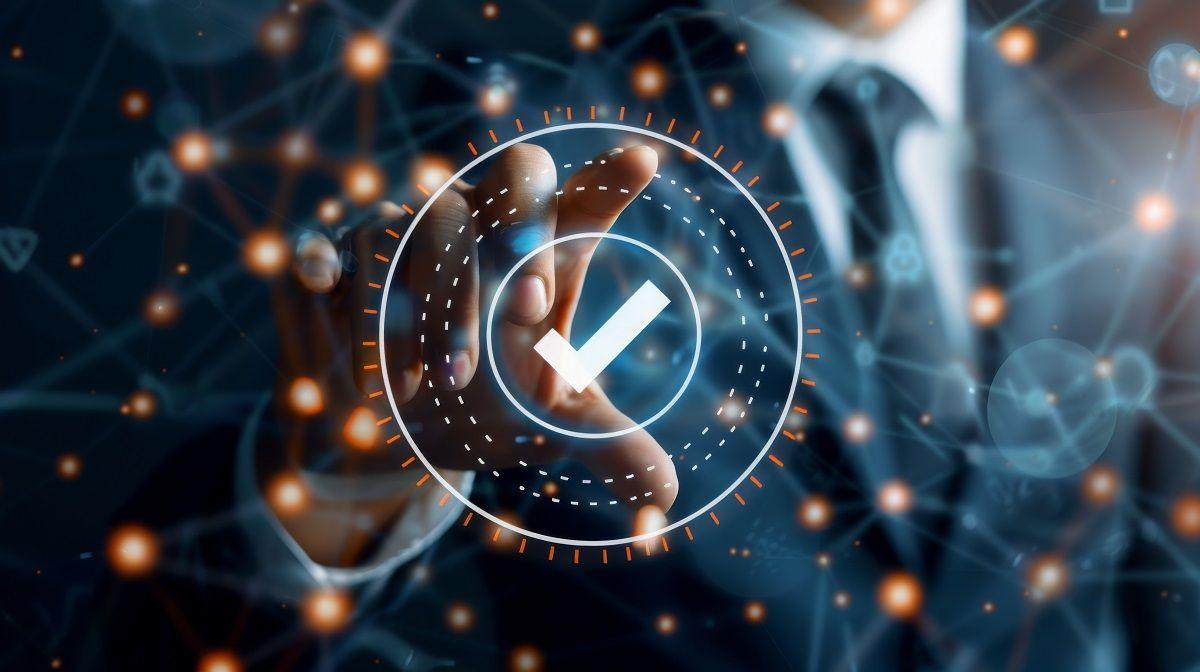
Measurable Impact
Since implementing the human-in-the-loop document automation solution, the insurance provider has experienced significant efficiency gains in their underwriting processes:
- 25% reduction in underwriting cycle times: By automating routine tasks and providing intelligent decision support, underwriters can focus on more complex cases, leading to faster turnaround times.
- 40% decrease in underwriting errors: The machine learning models, trained on expert-annotated data, can identify and flag potential errors or inconsistencies, improving the overall accuracy of underwriting decisions.
- Improved knowledge retention and scalability: The captured underwriting knowledge is now centralized and accessible, enabling better knowledge transfer and scalability as the organization grows.
By leveraging the power of human-in-the-loop machine learning and seamless workflow integration, the insurance provider has successfully addressed the challenge of underwriting knowledge retention, paving the way for more effective automation and continuous process improvement.